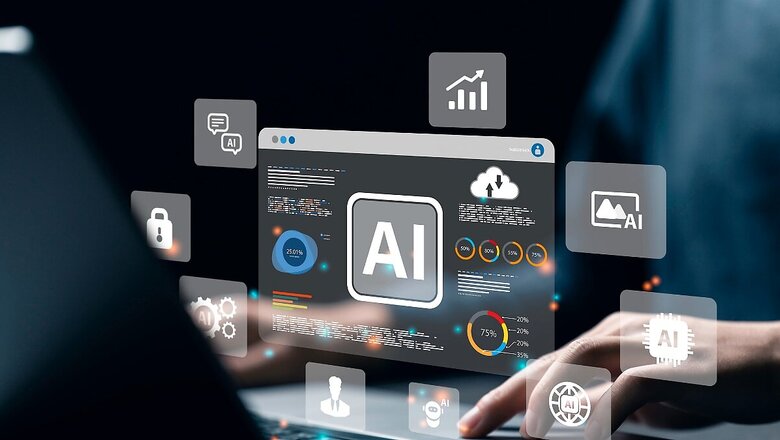
views
College hoops fans might want to think again before pinning their hopes of a perfect March Madness bracket on artificial intelligence.
While the advancement of artificial intelligence into everyday life has made “AI” one of the buzziest phrases of the past year, its application in bracketology circles is not so new. Even so, the annual bracket contests still provide plenty of surprises for computer science aficionados who’ve spent years honing their models with past NCAA Tournament results.
They have found that machine learning alone cannot quite solve the limited data and incalculable human elements of “The Big Dance.”
“All these things are art and science. And they’re just as much human psychology as they are statistics,” said Chris Ford, a data analyst who lives in Germany. “You have to actually understand people. And that’s what’s so tricky about it.”
Casual fans may spend a few days this week strategically deciding whether to maybe lean on the team with the best mojo — like Sister Jean’s 2018 Loyola-Chicago squad that made the Final Four — or to perhaps ride the hottest-shooting player — like Steph Curry and his breakout 2008 performance that led Davidson to the Sweet Sixteen.
The technologically inclined are chasing goals even more complicated than selecting the winners of all 67 matchups in both the men’s and women’s NCAA tournaments. They are fine-tuning mathematical functions in pursuit of the most objective model for predicting success in the upset-riddled tournament. Some are enlisting AI to perfect their codes or to decide which aspects of team resumes they should weigh most heavily.
The odds of crafting a perfect bracket are stacked against any competitor, however advanced their tools may be. An “informed fan” making certain assumptions based on previous results — such as a 1-seed beating a 16-seed — has a 1 in 2 billion chance at perfection, according to Ezra Miller, a mathematics and statistical science professor at Duke.
“Roughly speaking, it would be like choosing a random person in the Western Hemisphere,” he said.
Artificial intelligence is likely very good at determining the probability that a team wins, Miller said. But even with the models, he added that the “random choice of who’s going to win a game that’s evenly matched” is still a random choice.
For the 10th straight year, the data science community Kaggle is hosting “Machine Learning Madness.” Traditional bracket competitions are all-or-nothing; participants write one team’s name into each open slot. But “Machine Learning Madness” requires users to submit a percentage reflecting their confidence that a team will advance.
Kaggle provides a large data set from past results for people to develop their algorithms. That includes box scores with information on a team’s free-throw percentage, turnovers and assists. Users can then turn that information over to an algorithm to figure out which statistics are most predictive of tournament success.
“It’s a fair fight. There’s people who know a lot about basketball and can use what they know,” said Jeff Sonas, a statistical chess analyst who helped found the competition. “It is also possible for someone who doesn’t know a lot about basketball but is good at learning how to use data to make predictions.”
Ford, the Purdue fan who watched last year as the shortest Division I men’s team stunned his Boilermakers in the first round, takes it a different direction. Since 2020, Ford has tried to predict which schools will make the 68-team field.
In 2021, his most successful year, Ford said the model correctly named 66 of the teams in the men’s bracket. He uses a “fake committee” of eight different machine learning models that makes slightly different considerations based on the same inputs: the strength of schedule for a team and the number of quality wins against tougher opponents, to name a few.
Eugene Tulyagijja, a sports analytics major at Syracuse University, said he spent a year’s worth of free time crafting his own model. He said he used a deep neural network to find patterns of success based on statistics like a team’s 3-point efficiency.
His model wrongly predicted that the 2023 men’s Final Four would include Arizona, Duke and Texas. But it did correctly include UConn. As he adjusts the model with another year’s worth of information, he acknowledged certain human elements that no computer could ever consider.
“Did the players get enough sleep last night? Is that going to affect the player’s performance?” he said. “Personal things going on — we can never adjust to it using data alone.”
No method will integrate every relevant factor at play on the court. The necessary balance between modeling and intuition is “the art of sports analytics,” said Tim Chartier, a Davidson bracketology expert.
Chartier has studied brackets since 2009, developing a method that largely relies on home/away records, performance in the second half of the season and the strength of schedule. But he said the NCAA Tournament’s historical results provide an unpredictable and small sample size — a challenge for machine learning models, which rely on large sample sizes.
Chartier’s goal is never for his students to reach perfection in their brackets; his own model still cannot account for Davidson’s 2008 Cinderella story.
In that mystery, Chartier finds a useful reminder from March Madness: “The beauty of sports, and the beauty of life itself, is the randomness that we can’t predict.”
“We can’t even predict 63 games of a basketball tournament where we had 5,000 games that led up to it,” he tells his classes. “So be forgiving to yourself when you don’t make correct predictions on stages of life that are much more complicated than a 40-minute basketball game.”
Comments
0 comment