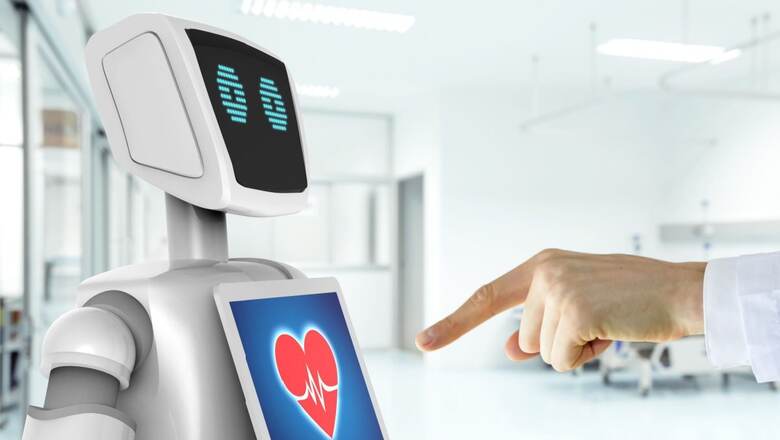
views
Chatbots and NLPs assist patients by swiftly analyzing medical data, symptoms, and history. They provide accurate insights, suggest potential conditions, and offer general advice. While not a replacement for doctors, they enhance healthcare accessibility, answer queries promptly, and guide users towards appropriate medical resources, improving patient engagement and initial assessments.
Applications of Chatbots and NLPs
“AI enabled chatbots or models ( like Med-PaLM2 or Hippocratic) have been found to be extremely capable of diagnosing ailments and providing medical advice, not to mention passing medical exams. And this shouldn’t come as a surprise because Generative AI models can not only “remember” millions of X-rays, medical reports, medical books but they can also understand and figure out the hidden patterns and information spread across the medical reports of a patient. Given that these models will become smarter and faster with every passing day, it is a foregone conclusion that they can do a good job in medical science. An AI system can be the best possible assistant to a doctor by suggesting the most probable path at every stage and more importantly pointing out the less probable ones,” says Pawan Prabhat, co-founder, Shorthills AI.
Large language Models like Chat GPT and PalM2 are poised to be the future game changers in healthcare. They can be the possible cure for some of the myriad problems plaguing the healthcare industry. “The application of technology in areas like interpreting images, developing new medicines, assessing retinal scans etc. are well known. But the usefulness of LLMs in direct patient care and dispensing medical advice may be a long distance away. After all, medicine is not only science but also an art. These models excel at referring to dense medical knowledge and applying it to the diagnosis in question. But patient care also involves customising advice to the individual patient with their unique set of medical and personal history, their physiology and co-morbidities and needs to be delivered in the patients social and cultural context. Besides, patients’ interpretation of the severity of their own disease and availability of resources for its management vary widely,” says Dr Preeti Goyal, Vice President, Medical Services, MediBuddy vHealth.
Upcoming NLPs
Large companies like Google have been working on using Generative AI for revolutionizing healthcare. In May month of this year, Google announced bold progress in AI, advancing Med-PaLM 2 for Healthcare. “Although Med-PaLM 2 is still being tested by select healthcare and science organisations, it has shown clinical answers of more than 90% Accurate. Med-PaLM 2 is the successor of Med-PaLM, which had already achieved more than passing marks (60+%) in on U.S. Medical Licensing Exam-style [USMLE] questions. Med-PaLM 2, Google’s next-generation Large Language Model, has been developed specifically to generate medical insights and it can answer questions and summarise insights from a variety of dense medical texts. Multimodal capabilities to synthesise information like X-rays and mammograms are now being integrated to achieve better patient outcomes. But we need to take this success with more than a pinch of salt,” says Paramdeep Singh, Co-founder, Shorthills AI.
Challenges
Generative models are essentially models that predict the next word based on the text that they have seen. Cracking exam style questions is a task they can do well, because they have seen that kind of material in the past and are generating text having seen that data. However, real life medical situation can be very different. There could be multiple symptoms that your doctor would be able to judge based on your physical examination, which Generative Models might miss. On top of that, Generative models hallucinate. That means, they might be generating answers that are not correct. “Large companies like Microsoft understand the legal risk attached with this as well. They have started putting guardrails on their popular applications like ChatGPT. Now if you ask for medical advice from ChatGPT, it returns back an answer saying that it is not a medical practitioner and cannot give medical advice. The regulations too in this space are also not crystal clear. Chatbots and NLP have demonstrated remarkable accuracy in solving some of language tasks related to medical information extraction. This can also be helpful for doctors, when they are trying to look for medical cases and trying to find solutions to complex cases. This intuitive interaction can bridge the gap between knowledge stored in medical journals/ dense books and the practicing doctor, enabling them to access accurate information and guidance promptly. In the future, this may also be helpful in assisting patients by streamlining the process of obtaining medical information and advice. These intelligent systems can comprehend and interpret natural language, allowing patients to communicate with them in a conversational manner, just as they would with a healthcare professional. However, till we have all the guardrails and regulations in place, it is best to rely for medical advice from an expert doctor,” adds Singh.
Despite the extremely promising capabilities displayed by AI in medical science, patients across the globe have been wary in accepting any diagnosis or treatment by an AI. Even if irrefutable proofs of the AI’s superior performance have been communicated to them, patients have been found to be more willing to accept treatment from a human being. One reason why they don’t believe that an AI can do a good job is simply because AI systems in the past have not been very accurate. Another more interesting and important reason is that all patients feel that they are “unique” and any AI system cannot possibly know about their constitution without knowing their full life history or without seeing them in person. People have been seeing doctors since ages and any new advancement in technology – MRI, X-Ray – is perceived to be an aid to the doctor rather than a substitution. Building this trust will be the deciding factor in how soon AI is adopted in medical-care because the capabilities of these AI systems have been proved beyond doubt,” adds Prabhat.
The challenge is also that the data used to develop a healthcare AI algorithm uses a specific dataset which is neither standard nor exhaustive. Therefore, the resulting data model may not be representative of real patient data in the local community and may be misleading at best and downright dangerous in the extreme. At best, the currently available generation of healthcare AI can assist clinicians by taking on some of their tasks before patient care is actually delivered.
“The use of healthcare AI in non-clinical settings is here and now, but their usefulness and safety in direct patient care is a long and tedious journey to be taken by technologists, clinicians, and scientists together with the regulators and governments,” signs off Dr Goyal.
Comments
0 comment